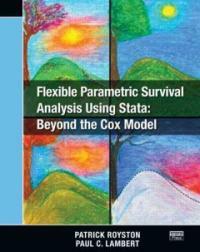
Flexible parametric survival analysis using Stata: beyond the Cox model
- 발행사항
- College Station, TX :,Stata Press,,2011
- 형태사항
- xxvi, 347 p. : ill.; 24cm
- ISBN
- 9781597180795
- 청구기호
- 413.8 R892f
- 서지주기
- Includes bibliographical references (p. [331]-339) and index
소장정보
위치 | 등록번호 | 청구기호 / 출력 | 상태 | 반납예정일 |
---|---|---|---|---|
이용 가능 (1) | ||||
1자료실 | 00015104 | 대출가능 | - |
- 등록번호
- 00015104
- 상태/반납예정일
- 대출가능
- -
- 위치/청구기호(출력)
- 1자료실
책 소개
Through real-world case studies, this book shows how to use Stata to estimate a class of flexible parametric survival models. It discusses the modeling of time-dependent and continuous covariates and looks at how relative survival can be used to measure mortality associated with a particular disease when the cause of death has not been recorded. The book describes simple quantification of differences between any two covariate patterns through calculation of time-dependent hazard ratios, hazard differences, and survival differences.
목차
Introduction
Goals
A brief review of the Cox proportional hazards model
Beyond the Cox model
Why parametric models?
Why not standard parametric models?
A brief introduction to stpm
Basic relationships in survival analysis
Comparing models
The delta method
Ado-file resources
How our book is organized
Using stset and stsplit
What is the stset command?
Some key concepts
Syntax of the stset command
Variables created by the stset command
Examples of using stset
The stsplit command
Conclusion
Graphical introduction to the principal datasets
Introduction
Rotterdam breast cancer data
England and Wales breast cancer data
Orchiectomy data
Conclusion
Poisson models
Introduction
Modeling rates with the Poisson distribution
Splitting the time scale
Collapsing the data to speed up computation
Splitting at unique failure times
Comparing a different number of intervals
Fine splitting of the time scale
Splines: Motivation and definition
FPs: Motivation and definition
Discussion
Royston?Parmar models
Motivation and introduction
Proportional hazards models
Selecting a spline function
PO models
Probit models
Royston?Parmar (RP) models
Concluding remarks
Prognostic models
Introduction
Developing and reporting a prognostic model
What does the baseline hazard function mean?
Model selection
Quantitative outputs from the model
Goodness of fit
Out-of-sample prediction: Concept and applications
Visualization of survival times
Discussion
Time-dependent effects
Introduction
Definitions
What do we mean by a TD effect?
Proportional on which scale?
Poisson models with TD effects
RP models with TD effects
TD effects for continuous variables
Attained age as the time scale
Multiple time scales
Prognostic models with TD effects
Discussion
Relative survival
Introduction
What is relative survival?
Excess mortality and relative survival
Motivating example
Life-table estimation of relative survival
Poisson models for relative survival
RP models for relative survival
Some comments on model selection
Age as a continuous variable
Concluding remarks
Further topics
Introduction
Number needed to treat
Average and adjusted survival curves
Modeling distributions with RP models
Multiple events
Bayesian RP models
Competing risks
Period analysis
Crude probability of death from relative survival models
Final remarks
References
Author index
Subject index