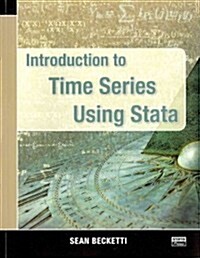
Introduction to time series using Stata
- 개인저자
- Sean Becketti
- 판사항
- 1st ed
- 발행사항
- College Station, Tex:,Stata Press,,2013
- 형태사항
- xxv, 443 p. : ill; 24cm
- ISBN
- 9781597181327
- 청구기호
- 413.8 B396i
- 서지주기
- Includes bibliographical references and index
소장정보
위치 | 등록번호 | 청구기호 / 출력 | 상태 | 반납예정일 |
---|---|---|---|---|
이용 가능 (1) | ||||
1자료실 | 00015090 | 대출가능 | - |
- 등록번호
- 00015090
- 상태/반납예정일
- 대출가능
- -
- 위치/청구기호(출력)
- 1자료실
책 소개
Recent decades have witnessed explosive growth in new and powerful tools for timeseries analysis. These innovations have overturned older approaches to forecasting, macroeconomic policy analysis, the study of productivity and long-run economic growth, and the trading of financial assets. Familiarity with these new tools on time series is an essential skill for statisticians, econometricians, and applied researchers.
Introduction to Time Series Using Stata provides a step-by-step guide to essential timeseries techniques?from the incredibly simple to the quite complex?and, at the same time, demonstrates how these techniques can be applied in the Stata statistical package. The emphasis is on an understanding of the intuition underlying theoretical innovations and an ability to apply them. Real-world examples illustrate the application of each concept as it is introduced, and care is taken to highlight the pitfalls, as well as the power, of each new tool.
Sean Becketti is a financial industry veteran with three decades of experience in academics, government, and private industry. Over the last two decades, Becketti has led proprietary research teams at several leading financial firms, responsible for the models underlying the valuation, hedging, and relative value analysis of some of the largest fixed-income portfolios in the world.
목차
Just enough Stata
Getting started
All about data
Looking at data
Statistics
Odds and ends
Making a date
Typing dates and date variables
Looking ahead
Just enough statistics
Random variables and their moments
Hypothesis tests
Linear regression
Multiple-equation modelsTime series
Filtering time-series data
Preparing to analyze a time series
Questions for all types of data
The four components of a time series
Some simple filters
Additional filters
Points to remember
A first pass at forecasting
Forecast fundamentals
Filters that forecast
Points to remember
Looking ahead
Autocorrelated disturbances
Autocorrelation
Regression models with autocorrelated disturbances
Testing for autocorrelation
Estimation with first-order autocorrelated data
Estimating the mortgage rate equation
Points to remember
Univariate time-series models
The general linear process
Lag polynomials: Notation or prestidigitation?
The ARMA model
Stationarity and invertibility
What can ARMA models do?
Points to remember
Looking ahead
Modeling a real-world time series
Getting ready to model a time series
The Box?Jenkins approach
Specifying an ARMA model
Estimation
Looking for trouble: Model diagnostic checking
Forecasting with ARIMA models
Comparing forecasts
Points to remember
What have we learned so far?
Looking ahead
Time-varying volatility
Examples of time-varying volatility
ARCH: A model of time-varying volatility
Extensions to the ARCH model
Points to remember
Models of multiple time series
Vector autoregressions
A VAR of the U.S. macroeconomy
Who’s on first?
SVARs
Points to remember
Looking ahead
Models of nonstationary time series
Trends and unit roots
Testing for unit roots
Cointegration: Looking for a long-term relationship
Cointegrating relationships and VECMs
Deterministic components in the VECM
From intuition to VECM: An example
Points to remember
Looking ahead
Closing observations
Making sense of it all
What did we miss?
Farewell